views
A Complete Guide to Healthcare Data Annotation Providers
Artificial intelligence (AI) is revolutionizing the healthcare industry, driving innovations in diagnostics, treatment planning, and patient care. However, a critical piece of the AI puzzle is often overlooked but indispensable: healthcare data annotation. Without accurately annotated data, training AI models capable of delivering reliable results in contexts such as medical imaging or patient monitoring would be nearly impossible.
This blog explores healthcare data annotation, the challenges it presents, how to select the right provider, and a roundup of the top players in the space. Whether you’re a healthcare provider, data scientist, or AI developer, this guide will help you understand the crucial components of choosing a healthcare data annotation provider.
What is Healthcare Data Annotation?
Healthcare data annotation involves labeling medical and healthcare data like images, charts, or patient records, so machine learning algorithms can learn from it. This includes annotating X-rays, MRIs, or CT scans, labeling text fields in patient reports, or categorizing symptoms and diagnoses.
For AI applications, such as identifying diseases in medical imaging or predicting patient outcomes, annotated data ensures algorithms are trained on high-quality datasets. But the process is highly complex due to the sensitivity and specificity of healthcare data.
Common Examples of Healthcare Data Annotation
- Medical Imaging: Labeling lung nodules in X-rays or segmenting tumor boundaries in MRIs.
- Electronic Health Records (EHRs): Annotating patient records to extract diagnoses, treatments, or demographic data.
- Symptom Analysis: Categorizing patient-reported symptoms for AI-driven diagnostic tools.
- Drug Development: Identifying biomarkers in datasets for improved drug discovery.
Regardless of the type, accurate healthcare data annotation is the foundation upon which reliable healthcare AI is built.
Challenges in Healthcare Data Annotation
Working with healthcare data is no simple feat. It involves navigating several challenges unique to this domain:
1. Data Privacy and Compliance
Healthcare data is highly sensitive and governed by stringent regulations such as HIPAA, GDPR, and other regional data protection laws. Annotation providers must abide by these regulations to ensure data security and compliance.
2. Domain-Specific Expertise
Precise annotation of healthcare data often requires domain expertise. Annotating CT scans or pathology slides, for example, may necessitate the input of radiologists or medical professionals.
3. Data Complexity
Healthcare data involves intricate patterns. For instance, distinguishing between different types of tissue in a medical scan requires significant attention to detail and specialized annotation tools.
4. Scalability
Training AI systems at scale requires vast amounts of annotated data. Scaling annotation teams while maintaining accuracy and compliance can be challenging and resource-intensive.
5. Cost
Annotation in healthcare tends to be more expensive compared to other industries due to its complexity, stringent accuracy requirements, and reliance on skilled labor.
Overcoming these challenges requires the expertise and resources of a quality healthcare data annotation provider.
Key Features to Look for in a Provider
With healthcare data annotation being so specialized, it’s critical to choose the right provider. Here are the features to prioritize:
1. Compliance with Regulations
Ensure the provider adheres to all applicable data protection laws, such as HIPAA or GDPR. Your data must remain secure and confidential throughout the annotation process.
2. Domain Expertise
Look for providers with teams that include trained medical professionals or radiologists capable of handling complex data annotations accurately.
3. Annotation Quality Assurance
Providers should implement robust quality assurance (QA) processes to verify the accuracy of annotations. Mistakes in healthcare data annotation could have serious consequences for AI model performance.
4. Scalability
If your project grows, make sure the provider can expand their services to handle larger datasets without compromising on quality.
5. Tooling and Technology
A good provider will leverage modern AI-powered annotation tools, ensuring efficient workflows, advanced capabilities like pre-annotation, and faster turnaround times.
6. Experience and Reputation
Research the provider’s track record. Look for case studies, reviews, and testimonials from other healthcare and AI organizations.
Top Healthcare Data Annotation Providers
While there are many annotation providers in the market, only a select few specialize in healthcare data. Here’s a look at some of the top players:
1. Macgence
Macgence excels in providing high-quality healthcare data annotations. Their resources include domain-specific experts who can annotate complex data such as radiology images and EHRs with precision. Macgence’s comprehensive solutions prioritize compliance, scalability, and QA, making them a top choice for healthcare projects.
2. Labelbox
Labelbox provides flexible annotation tools for handling large-scale medical datasets. Their platform’s intuitive interface allows for complex labeling workflows, including multi-object annotations common in medical imaging.
3. Scale AI
Scale AI specializes in providing annotation at scale, as the name suggests. They offer robust tools and expert annotators for medical data, making them ideal for larger AI projects.
4. CloudFactory
Known for their human-in-the-loop solutions, CloudFactory is skilled at combining expert annotators and advanced technology for accurate healthcare labeling.
5. Appen
Appen brings extensive experience and a global workforce to the table. They handle diverse datasets and offer annotation services designed to meet healthcare-specific needs, including compliance and scalability.
Case Studies and Success Stories
Macgence Boosts Training Accuracy for Radiology AI Solution
A radiology firm partnered with Macgence for annotating thousands of MRI datasets. By leveraging Macgence’s team of radiologists and domain experts, the firm improved their AI’s diagnostic accuracy by 15%, achieving better patient outcomes.
Labelbox Streamlines Annotation for Rare Disease Research
Labelbox helped a healthcare research lab annotate genetic datasets tied to rare diseases. Their advanced workflows shortened annotation times by 30%, accelerating insights into possible treatments.
Real-world examples like these show that selecting the right healthcare annotation provider can make or break an AI project.
The Future of Healthcare Data Annotation
The future of healthcare data annotation is promising, with several exciting trends on the horizon:
- Automated Annotation Tools: Pre-annotation and auto-labeling technologies are reducing the time required for manual labor, increasing efficiency.
- Collaboration with Medical Professionals: Annotators and medical specialists will continue to work in tandem, ensuring that annotations maintain clinical accuracy.
- Integration with Real-Time Data: Annotation of real-time data from IoT devices and wearables will open new possibilities for predictive healthcare AI systems.
- Privacy-Preserving Techniques: Advances in federated learning and differential privacy will make it easier to work with healthcare data without compromising patient privacy.
Organizations that adopt these advancements early will be well-positioned to harness the full potential of AI in transforming healthcare.
Start Building the AI-Powered Healthcare Systems of Tomorrow
Healthcare data annotation is the foundation for building sophisticated AI models that could redefine how we deliver care globally. By addressing the unique challenges of healthcare data and partnering with trusted providers like Macgence, organizations can unlock groundbreaking opportunities in diagnostics, treatment planning, and patient management.
Start your AI healthcare project the right way. Partner with expert annotation providers and set your AI models up for success.
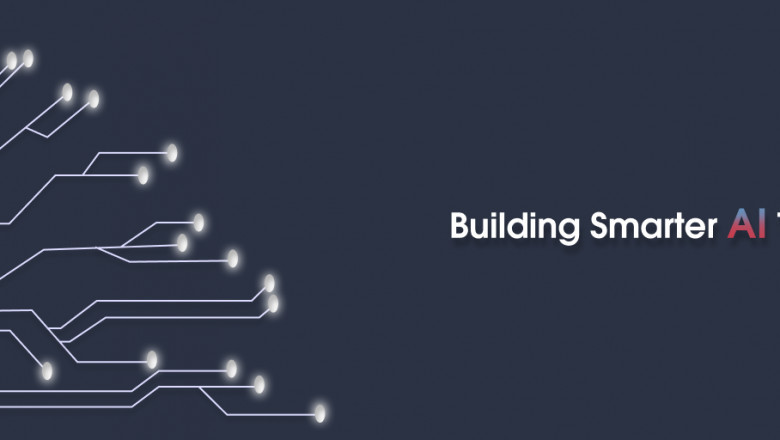

Comments
0 comment