views
The Crucial Role of Fairness Measures in AI Product Development
Artificial intelligence is fast changing our world and is transforming everything from the suggestions that we read online to the medical diagnosis we receive. As AI product development increases it is possible of these technologies to improve our lives is huge. But this power comes with a major obligation to ensure that AI technology is fair, and fair for every user.
Think of an artificial intelligence-powered system for loan applications that inexplicably rejects loans to those who belong to certain population groups. For instance, a facial recognition technology which performs much worse for people with darker skin tone. These aren’t just imagined scenarios, but real-world examples of how bias could be introduced in to AI systems, resulting in unfair outcomes and weakening trust.
This is when the importance of fairness measurements in the development of AI products becomes crucial. Integrating fairness concerns right from the beginning isn’t only an ethical necessity, but an essential aspect in building solid reliable, trustworthy, and trustworthy AI products.
The Concept of Bias in AI What is it? Where does it Originate?
Before we get into fairness metrics it’s important to know the root causes of bias within AI. Bias can be a factor in AI systems at different phases of development’s life cycle:
- Data Bias Data used to build AI models is usually an expression of social biases. If the data used to train depicts certain groups or reflects prejudgments from the past or historical prejudices, the AI model will most likely be able to learn and reinforce these biases. For example, if a hiring AI is based using historical data in which males were the majority in the top positions, it could bias favor male applicants.
- Algorithmic Bias: Even when the data appears to be neutral the structure for the AI algorithm itself could introduce bias. Certain algorithmic decisions or constraints could inadvertently favor certain groups over others.
- Sampling Bias: If the data used to train or test the AI model isn’t representative of the whole population that it serves and result in biased outcomes for those who are not represented.
- Measurement Bias The manner in the data is gathered and labeled could create bias. For instance, if sensors that collect data behave differently in different demographic groups, the data could be biased.
- Interpretation Bias Although the AI model’s output seems neutral, the way that humans interpret and respond to the output may create bias.
Reasons Fairness Measures are Not Negotiable when it comes to AI Product Development
Integrating fairness measures into the ai product development process is not just a “nice-to-have”; it’s a fundamental requirement for several reasons:
- Ethics: As creators of technological advancements, we are under an ethical responsibility to ensure that they do not perpetuate create more inequality in society. Making just AI systems is an issue of social justice.
- Legal compliance: Increasingly, regulations and legal frameworks are forming to combat discrimination and bias within AI systems. A proactive integration of fairness measures will help companies stay ahead of the changing legal regulations.
- building trust and Acceptance: Users are more likely to believe in and accept AI products if they are perceived to be impartial and fair. Unfair outcomes can result in damaging publicity or reputational injury and ultimately, hamper adoption.
- Enhancing Product Quality and Reliability AI systems that are biased tend to perform poorly for particular users, resulting in poor user experiences and diminishing the overall reliability and quality of the service.
- Inspiring innovation: By actively addressing issues of fairness, new product development services in the AI area can result in more inclusive and creative solutions that meet the needs of an array of users.
Exploring across the Landscape of Fairness Measures
The process of defining and evaluating the fairness of AI is a difficult and nuanced process. There isn’t one widely accepted definition of fairness because the most appropriate definition is dependent on the particular situation and the application. However, there are a few key fairness measures and concepts are widely employed in ai product development:
- Statistics Parity (Demographic Parity): This measure seeks in ensuring that the amount of outcomes that are positive is equal for all demographic groups. For instance, in the loan application system statistical parity means that the rate of acceptance of loan application is about the same for all races.
- Equal Chance: This metric focuses on ensuring that people from different groups with the same level of education have a similar chance of obtaining a positive result. In the context of hiring AI Equal Opportunity, it is the term used to describe how similarly qualified applicants from various genders are equally likely of being considered for interview.
- Equalized Odds This measure goes one step further than equal chance and is designed to ensure that people from different groups share the same true positive in comparison to the false negative rate. For a medical diagnosis AI has equal odds, this could mean that the AI is able to detect the presence of an illness (true positive) and to misidentify the presence of an illness (false positive) for various populations.
- calibration: A well-calibrated model assures that the predicted probability of an event corresponds to what actually happens to an event across various groups. For example, if a AI predicts 70% likelihood of defaulting for a particular group, then roughly 70% of people in that group are likely to default.
- Personal Fairness It is a principle that suggests people who are alike in the context in hand should be rewarded with similar results. Determining what “similar” is in a specific context is an essential aspect of applying fairness to each individual.
It is the AI Product Development Lifecycle with Fairness in Mind
Integrating fairness measures isn’t an isolated event; it is a continuous process throughout the entire Ai product development development life-cycle
- The Definition of the Problem and Setting Goals: Clearly define the issue that the AI product is aiming to address and determine any potential issues with fairness early. Think about the possible effects on various users.
- Data Processing and Collection Examine the data sources to identify potential biases. Implement strategies for data augmentation, re-sampling, or bias mitigation during preprocessing.
- Model Development and Selection Select algorithms and structures that are less susceptible to bias. Test different fairness constraints and regularization methods during training.
- Fairness Assessment: Rigorously evaluate the trained model by using appropriate fairness measures. Analyze performance disparities across different demographic groups.
- Bias Remediation and Mitigation: If biases are identified, you should implement mitigation strategies like adjusting parameters of the model, retraining using biased data, or employing techniques for post-processing to modify the predictions.
- Monitoring and Deployment: Continuously monitor the deployed AI system to ensure fairness and efficiency. Develop mechanisms for detecting and correcting any biases that are emerging in the real-world application.
- Clarity and Transparency: Try to ensure that AI is transparent during its decision-making processes. Explanable AI (XAI) methods will help users understand how the model makes its conclusions and help identify possible bias-causing factors.
The Function in AI Product Development Services and Agencies
Making fair and impartial AI products requires expert knowledge and a thorough knowledge about the AI creation and fairness concepts. Engaging with experienced ai product development services or an ai product development agency can be invaluable. These experts can provide guidance and support throughout the entire development process, helping you:
- Find out about fairness risks that could be a concern.
- Choose appropriate fairness measures and assessment techniques.
- Implement bias mitigation strategies.
- Make sure you are in compliance with applicable regulations.
- Create ethical and trustworthy AI product.
Selecting the best AI product development agency is essential. Choose a partner who has an knowledge about ethical AI principles, expertise in creating fair systems, and an unwavering determination to be transparent and accountable.
How Xcelore Can Help You Create Fair as well as Ethical AI Products
At Xcelore we recognize the importance of building a powerful AI along with the development of honest and ethical AI. As a leading provider of ai product development services, We are dedicated to assisting our clients in developing AI strategies that are not just ingenious but also fair and trustworthy.
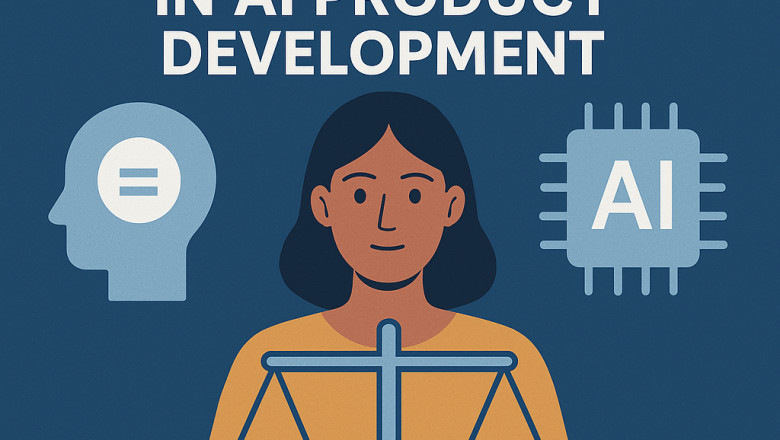

Comments
0 comment